The AI Technology Page:
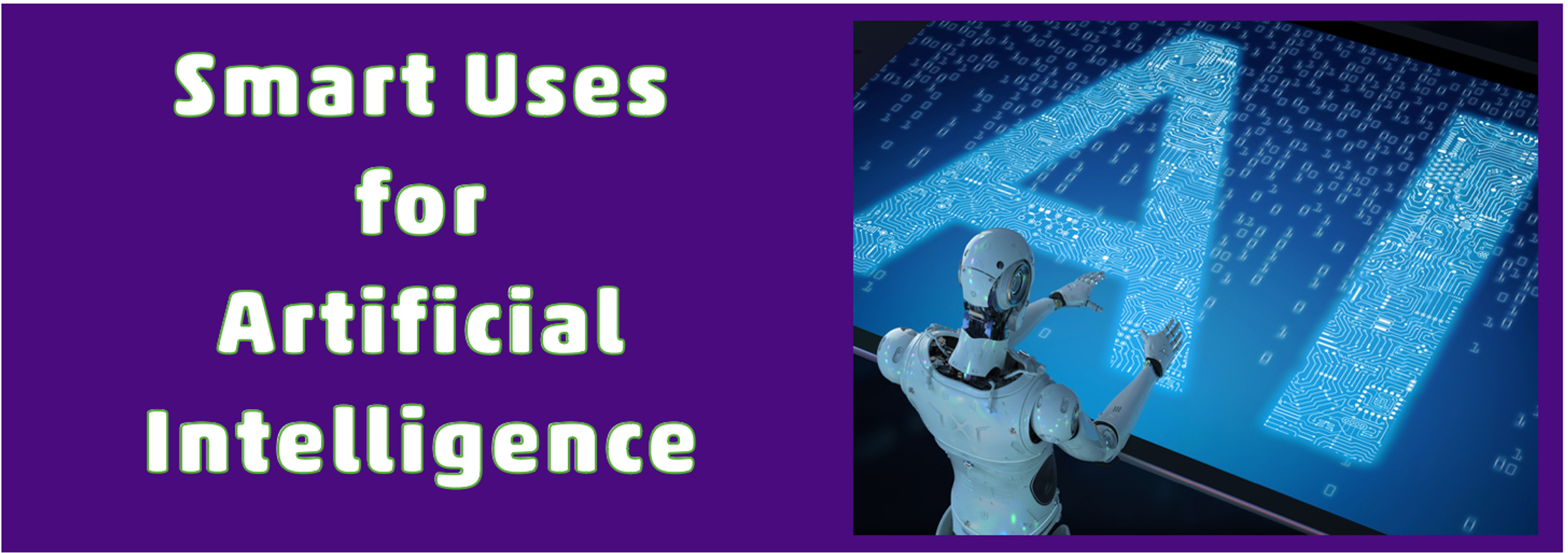
3 Key Differences Between Human and Machine Intelligence You Need to Know:
by Rafe Brena, Ph.D.
Despite all that has been said about AI capabilities (or often because of that), what AI can and can’t do is too often misunderstood. Indeed, not getting right what AI's real abilities are is a critical flaw that could undermine our interactions with AI. A few years ago, interacting with AI the right way could have been irrelevant for most people; now, it’s important for everyone, becoming more so as time goes by.
The advent of AI was marred by initial misconceptions –from humans, I mean. A notable example is the case of Blake Lemoine, a former Google engineer tasked with evaluating the Google AI chatbot LaMDA (a precursor to the current Gemini). Lemoine’s conversations with LaMDA impressed him so much that he deemed it ‘sentient’ and exhibiting ‘consciousness.’ I recall reading that he was reluctant to turn off LaMDA, fearing he would be ‘killing a soul.’ This incident occurred in May 2022, several months before the launch of ChatGPT.
Then, after ChatGPT was introduced in November 2022, many people were amazed at how easily the bot answered complicated questions, found information, and carried out tasks almost for free. But many didn’t get the bot’s tendency to make stuff up, with dangerous consequences.
One early casualty of “hallucinations” was that lawyer who relied on ChatGPT for an Avianca case, as reported by the New York Times in May 2023. “It did not go well,” comments the newspaper.
So, we really need to clearly understand what an AI conversational system can and can’t do. Perhaps the “can’t” part is more important.
Confusion about what AI does: two camps
There are two kinds of extreme positions about AI’s intelligence that are both misleading.
One is the camp of the overly AI optimists who believe AI will soon do “everything humans do.” Some call this “Artificial General Intelligence” (AGI for short). Though real scientists do not belong here, there are high-profile personalities like Elon Musk who see AGI systems arriving next year or so.
Look, I’ve been in AI since the 1980s last century (eighties, it’s not a typo), and I’ve seen loads and loads of unfulfilled AI promises, like “AI doctors” promised even before I was in AI (right now, Google is improving “Med-Gemini,” but we’ll have to wait and see whether it delivers on its promise).
The problem with outlandish promises is that they lead to disenchantment and budget cuts later on. This has been called “AI winters,” and it’s when nobody talks about AI outside specialized conferences. I was there; I saw it.
The opposite camp is the “AI denialists,” which is not the same as “AI pessimists” (true pessimists could think AI has real cognitive abilities, but those abilities will be used for evil).
AI Denialists think AI just regurgitates content from training data. They are wrong.
This idea of “regurgitation” implies that AI “doesn’t understand” what it’s saying. And this is the biggest misunderstanding about AI out there. The critical term here is “understands.” What do we mean by “AI understands”?
I wrote the post “Does ChatGPT Understand Or Not? I’ve Just Changed My Mind” a year ago. I recount there how I was convinced about a form of “understanding” by Sebastien Bubeck, researcher at Microsoft:
He said that it would be impossible to follow the instructions given by the user in the prompt without understanding them. Touché.
This argument is easily verifiable with an experiment. Of course, we are not going to ask the machine “Do you understand?” but instead, we are going to ask a question so that the answer can be checked by us.
Consider the following prompt:
I need to find an acronym for the “Society for Good Deeds Promotion.” Start with the string “Society for Good Deeds Promotion” and remove letters and spaces until the acronym is found. Please don’t add any other letter, just remove from the original title.
Microsoft’s Copilot came up with the solution “SOGODEP,” which could be a catchy acronym or not, but does respect the rules I gave.
Tell me this: what are the odds that “just by chance” (or, more technically said, “by probabilistic plausibility”) the chatbot comes up with the answer “SOGODEP”?
Practically none. Zero. The bot has to understand what the request means.
So we can safely conclude Copilot “understood” my request. I call this kind of machine comprehension “behavioral understanding” to differentiate it from the humans’ “experiential understanding” (more on this below).
By the way, Google’s Gemini proposed the acronym “SFGDP” for the society, simply taking the first letter of each word. It was lazy, but it did comply with the request.
Now, we are ready to check three differences between human and machine intelligence.
Difference 1: Humans have feelings; machines pretend to have them
Some folks believe that logical problem-solving intelligence is the only edge we humans have when facing situations, but it’s not: from the evolutionary perspective, feelings have played at least an equally important role.
When a caveman faced the decision of whether to face a possibly dangerous animal or run away, the decision was not taken by a deductive process: he’d feel either fear or a rush of courage and adrenaline, and that was it. Individuals with badly tuned feelings (for example, those reckless with lions) would perish (in the case of too much fear, they’d be undernourished, as they would fail to attack suitable prey).
So, having, expressing, and interpreting the feelings of others is in our DNA. That’s why “emotional intelligence” means something at all.
But things get tricky when somebody or something dupes us with apparent signals of feelings. That’s what (good) actors do. That’s also what very sophisticated machines do.
Have you heard GPT-4o talking voice? If you haven’t, run to this link and check the amazing range of emotions the chatbot’s voice shows. From happy to intrigued to flirty, the GPT-4o voice has nothing of the robotic voices we heard from machines of yore. It’s a voice with emotion… except that, as we mentioned above, a chatbot doesn’t have feelings.
What tech companies have done instead is to build machines that pretend to have feelings. This is entirely feasible.
The capability to pretend to have feelings has been naturally incorporated into chatbots as a result of the huge volumes of data they ingested from human sources during the training phase: most humans generating the texts and voices used for training had, indeed, real emotions.
When we, humans, hear a voice expressing emotion, we immediately get hooked. It’s a reflex. We are hardwired to pick up emotions.
Machines are getting better and better at pretending to have emotions, and we have to get used to this new reality instead of getting duped like Blake Lemoine was a couple of years ago.
Now that machine voices are not “robotic” anymore, lack of expressivity isn’t a way of telling people apart from robots.
Machines are trained to dupe us when they pretend to have feelings, and they are getting too good for our own sake.
Difference 2: Humans either understand or don’t. This is a big one.
We humans have the “experience of understanding,” that is, we feel something when we understand something we didn’t previously.
I remember distinctly when, while in high school, I understood Hardy’s definition of a limit in Calculus. It was like something suddenly made complete sense. That’s why it’s represented in cartoons as an electric bulb lighting up.
But machines don’t work this way.
I already discussed above what a “behavioral understanding” of machines is and how it differs from humans’ “experiential understanding.” In short, the first one is about doing things that are impossible to do unless we understand what we are talking about, while the second one is the light bulb we feel in our heads.
Many would consider experiential understanding like “true understanding,” but that’s unfair to machines because they have no feelings whatsoever, and experiential understanding is ultimately the feeling of understanding.
The most important realization I had about behavioral understanding is that it can be measured. Yes, with banks of tests, we can count how many questions the machine gets right and wrong.
Many tests are directly related to understanding, like the WinoGrande collection, which is focused on commonsense reasoning. Personally, I’ve been interested in commonsense reasoning because it’s an area particularly hard for machines and easy for humans.
Take, for instance, the following question, typical of WinoGrande:
The trophy didn’t fit in the suitcase because it was too big. What was too big?
a) The trophy
b) The suitcase
Questions like this are trivial for people but not for machines. There is a wealth of assumptions that we effortlessly make about suitcases and other objects in the physical world (like “suitcases hold things inside them, not outside”) that are not “natural” for machines that haven’t lived in that physical world.
Measuring understanding in machines (through test banks) means that improvements in understanding can be objectively demonstrated. So, higher intelligence in machines doesn’t mean any longer they are magically “getting” an idea, but instead, they are gradually inching to better and better scores in the cognitive tests.
Difference 3: Machines don’t hesitate
Some of us are puzzled by the assurance that chatbots show when answering our queries, oftentimes only to find out that the response was wrong.
I’m tired of explaining to Gemini, Claude, or Copilot why they were wrong, only to get a meaningless polite apology like, “I appreciate you letting me know that my answer was wrong.”
If they were not sure about the answer, why then they present it so confidently?
Well, because this is a bad way of framing the question.
You see, “to be confident” is a feeling, and as we saw in difference #1, machines don’t have feelings, so they can’t be confident or insecure.
So, we humans are the ones to blame for taking the machine’s prose as “confident.”
A different point is whether we can adjust machines to show, in some cases, a lack of confidence because, in reality, not every time machines have the same degree of certainty.
For instance, last week, I was reviewing the output of a neural network image classifier for a toy example (the CIFAR-10 dataset; see reference below). The output had the “one-hot” format (one output for every class in the classifier), and values were probabilities, as follows for one of the images:
airplane: 0.002937
automobile: 0.875397
bird: 0.001683
cat: 0.007123
deer: 0.000186
dog: 0.003424
frog: 0.002319
horse: 0.000454
ship: 0.002345
truck: 0.104132
Of course, the system chooses “automobile” for this particular image (the maximum probability), but we can see that its probability was not 100% but slightly less. Moreover, the “truck” class had a not negligible probability hundreds of times higher than the “horse” answer.
But in cases like this, the system is not going to say, “Um, most likely it’s a car, but it could also be a truck.” When we reduce the answer to “automobile,” we are discarding the rich information about the likelihood of each alternative.
From the technical point of view, it would be entirely possible to reflect likelihoods in machines’ answers, but it hasn’t been done so far. I personally think more “insecure” machines would be less puzzling and more relatable for us, so their interactions with us would be more natural.
Closing thoughts
We humans never in history had to deal with an alien form of intelligence. Even when dealing with intelligent animals (like chimps, who are able to beat children in some puzzles; see reference), these ones share too much with us in order to feel like an “alien” intelligence.
AI chatbots (and even worse in the case of the latest voice assistants, like GPT-4o) took us by surprise, and many didn’t know what to make of the strange abilities of the new machines.
Instead of treating advanced bots as just tools, we often projected our own cultural biases, preconceptions, and even fears onto them, anthropomorphizing them to make them palatable. But in doing so, we dressed them in clothes that didn’t really suit them, as if we were dressing a monkey in a suit.
References
Cukierski, W., 2013. CIFAR-10 — Object Recognition in Images.
Kaggle. https://kaggle.com/competitions/cifar-10
Sakaguchi, K., Bras, R.L., Bhagavatula, C. and Choi, Y., 2021. Winogrande: An adversarial Winograd schema challenge at scale. Communications of the ACM, 64(9), pp.99–106.
Horner, V. and Whiten, A., 2005. Causal knowledge and imitation/emulation switching in chimpanzees (Pan troglodytes) and children (Homo sapiens). Animal cognition, 8, pp.164–181.